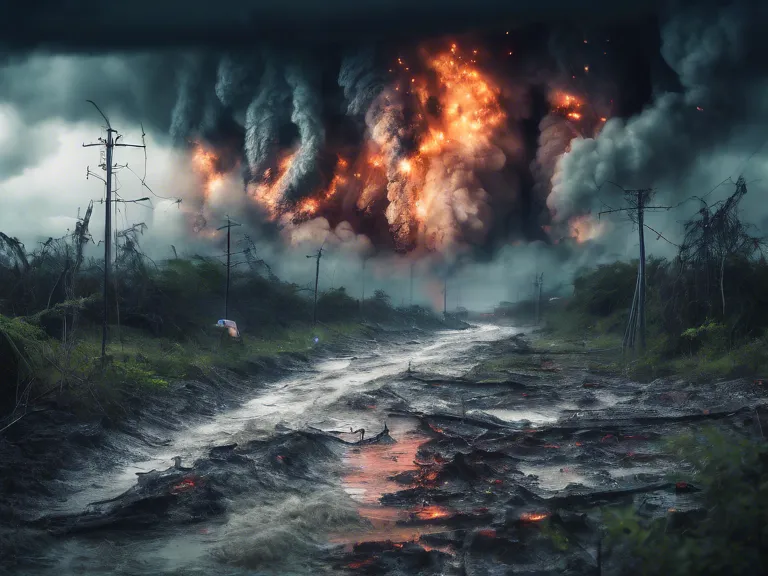
Data science plays a crucial role in forecasting environmental disasters by analyzing large amounts of data to predict natural events such as hurricanes, earthquakes, and tsunamis. Using various techniques and algorithms, data scientists can identify patterns and trends in environmental data that can help in predicting and preparing for potential disasters. This article explores the importance of data science in forecasting environmental disasters and how it can help save lives and mitigate the impact of catastrophic events.
One of the key ways that data science helps in forecasting environmental disasters is through predictive modeling. By collecting and analyzing historical data on past disasters, data scientists can create models that can predict the likelihood and severity of future events. These models can take into account various factors such as weather patterns, geological data, and human activities to generate accurate forecasts.
Data science also plays a role in monitoring and early warning systems for environmental disasters. By continuously collecting and analyzing real-time data from sensors and satellites, data scientists can detect precursors to disasters and issue timely warnings to at-risk populations. This rapid response can help in evacuating people, securing infrastructure, and reducing the impact of the disaster.
In addition to predicting and monitoring disasters, data science is also essential in analyzing the aftermath of disasters. By examining data on the damage caused by disasters and the response efforts, data scientists can identify areas for improvement and develop more effective strategies for disaster preparedness and response in the future.
Overall, data science is a powerful tool in forecasting environmental disasters and mitigating their impact. By harnessing the power of data and technology, we can better understand natural processes, predict disasters, and save lives. As the world faces increasing environmental challenges, the role of data science in disaster forecasting will only become more critical.